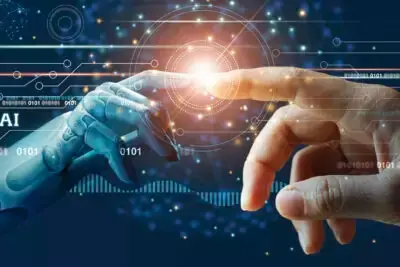
25 Sep 2021
AI-powered applications are radically transforming other medical services, including breast cancer screening and are aiding experts rather than replacing them. With breast screening, the AI software detects and displays suspicious features in the image, and predictive software helps clinical diagnosis.
Expertly trained dosimetrists in the radiation therapy world have expressed a view that Artificial Intelligence (AI) may be taking away a part of their job that requires an expertly trained eye: organ-at-risk contouring. In some clinics, manual contouring is being substituted with autocontouring, hoping that it saves time and improves accuracy and consistency. Does this inevitably mean that AI will take the valued dosimetrist role?
What’s the problem?
Innovation and advancements to better our lives is a hope and a theme that spans human existence. However, it often comes at a price and has consequences. The industrial revolution saw the manual effort of farming turned to machine automation, and skills displacement inadvertently resulted. In hindsight, skillsets were re-directed, but we still need to ask the question: just because technology can replace humans, should it? The health sector demands accuracy, timely treatment, and efficiency without sacrificing quality and personal care. The 2019 Topol Review Preparing the healthcare workforce to deliver the digital future (UK) provides a breakdown of the evolution and future of clinical practice with emerging technologies. Amongst their independent review, it states that ” The patient must be considered to be at the center when assessing and implementing any new technologies” (p6, Topol, 2019).
The question therefore is, does AI provide benefits to the patient? Not directly. What part does AI play within radiation therapy, and is it just taking jobs away from clinical experts that need to be exacting, consistent, and mindful of patients’ individual uniqueness?
A form of AI technology known as Deep Learning autocontouring has seen an increase in use for organ at-risk (OAR) delineations, replacing early parts of manual contouring, but leaving the quality assurance checks to be completed by expert clinicians. Dosimetrists in the radiation therapy world have raised concerns. This task is critical to ensure that healthy organs are spared from radiation, minimizing long-term health concerns and patient outcomes, and requires an expertly trained eye.
What is autocontouring?
Two forms of autocontouring currently exist. Atlas-based contours and Deep Learning. Atlas-based autocontouring uses approximately 10-20 anonymized contours from clinics and uses these as a base to build a solution to autocontour. (See An evaluation of atlas selection methods for atlas-based automatic segmentation in radiotherapy. 2019, Schipaanboord B et al. for more information) Deep Learning contouring is a form of Artificial intelligence based on neural networks mimicking the human brain. At Mirada Medical, the first AI clinically available AI Model, large anonymized data sets of more than a hundred are received from a clinic. These are then outlined by in-house dosimetrists, who curate and consistently contours the clinical datasets. An algorithm is trained to learn from this, forming the basis of Deep Learning’ models’ used in the clinics to contour new data. This technology is where the name DLCExpert originated. The keystone to any good model is well-curated and robust data sets. Mirada now offers an AI library of contours that covers the four anatomical regions: head and neck, thorax, prostate, and breast.
AI: Adversary or assistant
AI-powered applications are radically transforming other medical services, including breast cancer screening, and are aiding experts rather than replacing them. With breast screening, the AI software detects and displays suspicious features in the image, and predictive software helps clinical diagnosis. Yasmin McQuinlan believes that AI autocontouring is an assistant to clinical experts. She splits her time between Guys‘ NHS Hospital, London, and as an in-house research dosimetrist at Mirada. She believes that AI can minimize the perennial contouring problem of time to contour and reduce clinician inter and intra–observer variation that causes inconsistency of contours. It is about doing things slightly better for the patient.
University of New Mexico Cancer Center (2020) clinical evaluation of DLCExpert, found they saved 75% of the time in their head and neck contouring workflow.
Yasmin states that the time to manually contour complex head and neck anatomy can sometimes take between an hour and 90 minutes. With AI autocontouring, the initial manual effort is taken away. The automatic contours are created and land at the workstation within minutes after the patients are scanned. This efficiency allows the expert to do a thorough review of the delineations and essential quality checks. The dosimetrist can spend more time on complex cases and, eventually, enable clinics to reduce the time to treat patients. Yasmin believes the experts act as gatekeepers to their clinic’s standards; thus, AI is just a valuable assistant.
An independent AI autocontouring solution creates optimization and standardization of contours’ consistency. The lack of such consistency, according to Yasmin, “can impact the way we plan and evaluate these patients.”
This consistency and standardization issue is reported in a paper published by TROG for a head and neck trial, which showed the variation in target contouring contributed to poorer outcomes for patients (Peters et al. 2010).
In conclusion, the answer to ‘Will AI take my job’ from the perspective of this short article is no: AI is an assistant. If you would like to see if you can tell the difference between a human manual contour Vs a machine, take the Turing test. We will love to hear from you.
You may also like:
Take the Turing test
Comparative evaluation of autocontouring in clinical practice: A practical method using the Turing test Find out more about Deep Learning: White Paper on Deep Learning in Autocontouring
Watch the discussion on a webinar discussing: AI Adversary or Assistant
References:
The Topol Review: Preparing the healthcare workforce to deliver the digital future
Use of AI in Breast screening: https://www.nice.org.uk/advice/mib242/chapter/The-technologies
An evaluation of atlas selection methods for atlas-based automatic segmentation in radiotherapy. 2019, Schipaanboord B et al.
Peters, L. J., O’Sullivan, B., Giralt, J., Fitzgerald, T. J., Trotti, A., Bernier, J., … & Rischin, D. (2010). The critical impact of radiotherapy protocol compliance and quality in the treatment of advanced head and neck cancer: results from TROG 02.02. Journal of clinical oncology, 28(18), 2996-300
Comentarios