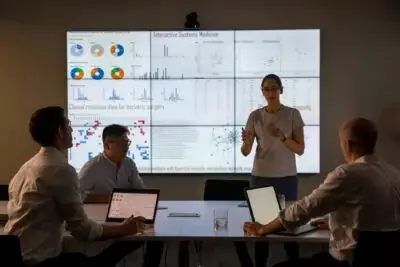
25 Sep 2021Guide to avoiding pitfalls when purchasing and implementing AI auto-contouring.
In 2018, Mirada Medical launched DLCExpert, the first AI clinical auto delineation tool for radiation oncology workflows. Mirada Medical has since gained valuable insights into how clinics can leverage the full benefits of AI autocontouring and avoid some of the problems and pitfalls which can arise when implementing any new technology.
This article highlights these pitfalls to help clinics looking to implement autocontouring in their radiation oncology workflow and ask the right questions in good time to gain all of the benefits while avoiding any problems.
AI autocontouring competition: From zero to 10+ in 3 years
In 2017, the number of search results on Google Scholar for the term ‘Radiation Oncology’ with ‘Artificial Intelligence’ or ‘Machine Learning’ stood at approximately 200 (see figure 1). This figure rose dramatically to 1200 by 2018 (Jarrett D et al. 2019). Despite the literary interest in 2018, only one clinical solution of AI, Deep Learning Organ at-risk delineation, was available – Mirada’s DLCExpert. Today, the use of AI applications are growing across the health industry, as is the number of AI autocontouring products and providers.
This rise in competition is great news for innovation and customers, but what has been learnt to date?
Journal papers like Liesbeth, Vandewinckele, et al provides an “Overview of artificial intelligence-based applications in radiotherapy” Radiotherapy and Oncology (2020). This paper provides clinical assessments and insights into the clinical implementation and quality assurance of these techniques to assist those hospitals interested in AI. From its vantage point as a first-mover, Mirada has also identified a list of key focus areas that, if not taken into account of, can result in sub-optimal implementation and reduced benefits such as efficiency, consistency and accuracy.
Key focus areas:
Configuration & algorithms – choose the right solution:
There are different approaches to autocontouring. Until recently, the most common underlying technology was “Atlas”. Today, Atlas technology is being replaced by superior AI-based Deep Learning technologies. Both solutions deliver autocontouring, but Deep Learning technology is increasingly being shown to deliver more usable clinical contours.
The difference – Atlas vs Deep Learning autocontouring:
Atlas-based autocontouring uses a combination of previously contoured anonymized patients, generally 20+, and a matching algorithm selects the patient or contour most similar to today’s patient before deformable image registration is used to transfer the contours from the Atlas onto the patient.
Deep learning uses hundreds of past anonymized cases (which have been carefully selected to ensure high-quality consistent input data) to train an artificial intelligence interface which learns how to predict the same structures onto new data. Deep learning contouring is a form of artificial intelligence based on neural networks mimicking the human brain.
Considerations:
Be sure to choose a solution that uses the optimal algorithm for you. More and more studies are showing that Deep Learning techniques outperform Atlas techniques. For some basic applications, Atlas may be sufficient, but for most clinical contouring in radiation therapy, we strongly recommend choosing a Deep Learning option (Mirada’s automation platform, WorkflowBox, supports both).
When choosing a Deep Learning option, take care to choose a system where the vendor can supply information about the number and quality of the original training datasets used.
Always select a system that allows you to test your data and see the output quality before you purchase. The input data can affect the output results
Has the system been independently validated? Ensure that it has and that you can obtain user references and peer reviewed papers.
System adaptability – one size does NOT fit all The ability to configure output structures and structure sets is another essential aspect that most hospitals will want to ensure that they have.
AI autocontouring providers should have the ability to refine and configure system outputs to meet your local or network protocols. At Mirada, our clinical experts will take your feedback and fine tune the output from the AI to your needs in order to follow your guidelines and best practice.
Avoid systems that are “one size fits all”. These systems may not deliver structure sets that conform to your needs
Vendor–neutral (or agnostic) systems will deliver better contour consistency
Many large equipment vendors are starting to build contouring into their hardware offerings. These, by definition, cannot be vendor agnostic. Therefore, within any department or network of providers where equipment from multiple manufacturers is used, each will output contours conforming to that manufacturer’s specification. There will be little or no consistency across outputs.
By contrast, vendor-neutral AI contouring specialists such as Mirada Medical can offer one consistent source of autocontouring that can be situated centrally and interoperate with each vendor system independently. With a single independent contouring solution, there is one source of consistent contours and, if required, a separate independent check other than the scanner, TPS or linac. All Mirada software delivers vendor neutrality. Mirada recognizes clinics already have multiple systems and we can offer seamless integration and interoperability.
Save time with AI-automation and remember, true automation means “Zero-click“ An AI application should work alongside your current workflows and add very few additional operator–steps as possible into your existing setup. The ideal is no extra steps. At Mirada, we call this “Zero-click“. It comes as standard due to our powerful automation platform that powers DLCExpert AI-autocontouring. Beware of vendor solutions that require additional manual input over and above your current workflow as these will be harder to integrate and will be less popular with staff.
Thinking about the future – choose a brand you can trust
Work with a company that has a long track record of providing excellent customer service and error-free performance. There are many new start-ups operating in the AI space – can they provide references from customers who have used them over many years?
Mirada is a brand that will grow with you. Innovation never stops, but it starts with your feedback. Choose a company that has a history of innovation to partner with, a brand with a track record of bringing not one, but many new inventions into everyday clinical use
Choose a Customer Success team you trust. For a busy clinical RT department, your software solutions are only as good as the training, support, and service you receive. At Mirada, our CS team will ensure the smooth running of DLCExpert all day, every day. You can rely on us to be ready when you need us, and in the unlikely event that you experience problems, we will be on hand to swiftly resolve these.
If you want to see how DLCExpert can serve your Radiation Therapy department, please book a demo. We’ll arrange this at your convenience. Figure 1. The number of search results by year for publications relating to “Radiation Oncology” and “Artificial Intelligence” or “Machine Learning”. Results from Google Scholar may represent a wider cross-section of publications than from PubMed. AI, Artificial Intelligence; ML, machine learning. © 2019 The Authors. Published by the British Institute of Radiology under the terms of the Creative Commons Attribution 4.0 Unported License http://creativecommons.org/licenses/by/4.0/, which permits unrestricted use, distribution and reproduction in any medium, provided the original author and source are credited. DLCExpert Validation Papers Assessment of manual adjustment performed in clinical practice following deep learning contouring for head and neck organs at risk in radiotherapy https://www.sciencedirect.com/science/article/pii/S2405631620300658 – Clinical Evaluation of Deep Learning and Atlas-based auto-contouring of bladder and rectum for prostate radiation therapy https://www.sciencedirect.com/science/article/pii/S1879850020301612 External validation of deep learning-based contouring of head and neck organs at risk https://www.sciencedirect.com/science/article/pii/S2405631620300312 – A prospective clinical evaluation of Mirada DLCExpert auto-contouring for head and neck OARs https://www.estro.org/Congresses/ESTRO-2020/380/ph-implementationofnewtechniques1/617/aprospectiveclinicalevaluationofmiradadlcexpertaut-. Evaluation of measures for assessing automatic OAR delineation acceptability and time-savings https://www.estro.org/Congresses/ESTRO-2020/461/physicstrack-imagingacquisitionandprocessing/1471/evaluationofmeasuresforassessingautomaticoardeline – Evaluation of measures for assessing time-saving of automatic organ-at-risk segmentation in radiotherapy https://www.sciencedirect.com/science/article/pii/S2405631619300636 – Clinical evaluation of Atlas and Deep Learning based automatic contouring for lung cancer https://www.sciencedirect.com/science/article/pii/S0167814017327299 Comparative evaluation of auto-contouring in clinical practice: A practical method using the Turing test https://aapm.onlinelibrary.wiley.com/doi/abs/10.1002/mp.13200 References: https://www.birpublications.org/doi/pdfplus/10.1259/bjr.20190001 Review article applications and limitations of machine learning in radiation oncology, Jarrett D, Stride E, Vallis K, Gooding MJ. Applications and limitations of machine learning in radiation oncology. Br J Radiol 2019; 92: 20190001 Overview of artificial intelligence-based applications in radiotherapy: Recommendations for implementation and quality assurance. Liesbeth Vandewinckele a,b,1, Michaël Claessens c,d,1, Anna Dinkla e,1,⇑, Charlotte Brouwer f, Wouter Crijns a,b, Dirk Verellen c,d, Wouter van Elmpt, Radiotherapy and Oncology 153 (2020) 55–66
コメント